Hypothesis Testing Is Right
10/2/21
Recently, Briggs, the krakenstician to the stars, named so for contributing his analysis to Sidney "release the kraken" Powell and used to help bolster the false claims of 2020 election fraud (spoiler: her contributed analyses and arguments were obliterated by the court) wrote More Proof Hypothesis Testing Is Wrong & Why The Predictive Method Is The Only Sane Way To Do Statistics where he continues his attempt to prove the most used statistical method in the world, hypothesis testing, to be false. Here I show that not only is his understanding of hypothesis testing incorrect, but his argument is self-defeating because hypothesis testing correctly used in fact helps to detect and correct the issue of overfitting he discusses.
First, his talk of probability being a real property of an object or not, etc., is a very interesting, but irrelevant distraction here. If I keep track of times a 6 lands on a dice, but then I clip off a corner of the dice, the frequency of observing a 6 will change. Or if I tilt a quincunx or Galton board, the distribution of the balls cascading down will change. So physical can be one part of probability, but probably not the whole story since we all use models too to simplify the world, which are not physical.
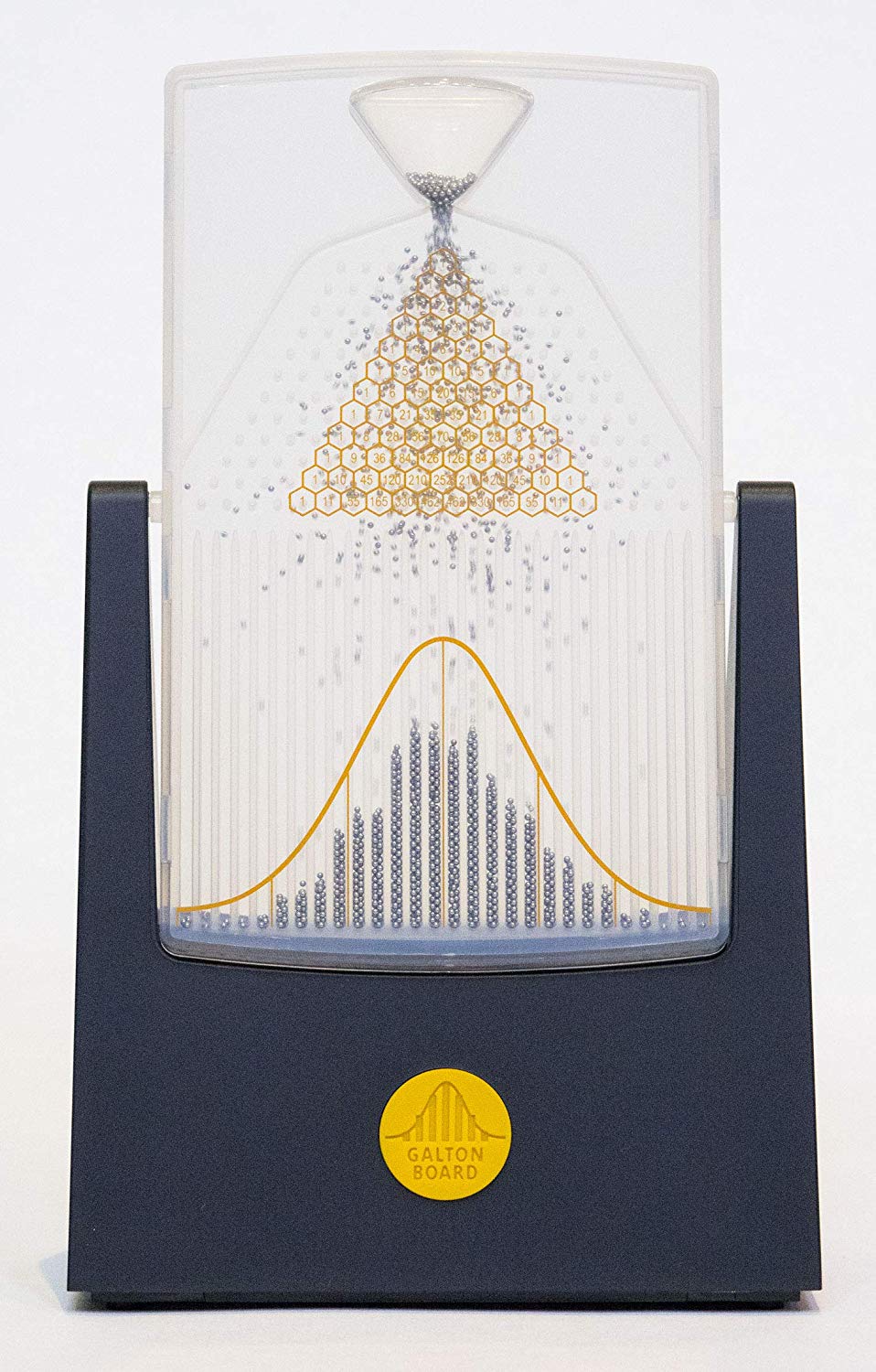
Get your quincunx or Galton board here
He discusses the interesting paper Real numbers, data science and chaos: How to fit any dataset with a single parameter by Boué. This paper doesn't mention hypothesis testing at all. Also, note that the α mentioned in the paper is not the same α as in hypothesis testing, which is P(rejecting H0|H0 is true) or the probability of making a Type 1 error. What the paper does do, is discuss a way to use a specific function to encode a dataset. Quoting the paper:
As should be clear by now, the parameter α is not really learned from the training data as much as it is explicitly built as an alternative (encoded) representation of Xtrain itself. In other words, the information content of α is identical (i.e. no compression besides a tunable recovery accuracy determined by α) to that of Xtrain and the model serves as nothing more than a mechanistic decoder.
In other words, they are essentially making Xtrain be α. The model is not being "trained" in the usual sense of the word.
Of course, there is nothing mysterious about hypothesis testing, α, or p-values at all as the krakenstician suggests. Let's say we are doing an experiment to see if a coin is fair or not. Basic human reasoning will introduce the need for something like α. Why? Because α can be thought of to be a formalization of the number of heads observed for which you start to conclude the coin is probably not fair. So focusing on the distance what you observe is from what you expect under a model makes good, logical sense.
Continuing on with the coin example, I'd expect to observe around 50 heads if the coin were fair. Say we do that experiment three times, and observe 92, 87, and 90 heads. From this evidence we conclude the coin is not fair (we reject a fair coin model). If instead we observed 55, 47, and 57 heads, we conclude the coin is fair (we do not reject a fair coin model). Could we be wrong with either conclusion? Of course. Briggs and the readers of his blog will not (cannot?) directly answer the very simple question "is the coin fair?". Doing so would admit hypothesis testing works.
Briggs writes
the null that α = 0 will always be slaughteredthe model will always predict badly
the fit says nothing about the ability to predict
However, these are not true in any universal general sense as he is implying, since we often find H0s that do not get rejected, find models that don't always predict badly, and find fits that do say something about an ability to predict. Of course, such models based on data and experiments and actually open to be falsified seem to do better than religion's track record of understanding and making predictions about the world.
Briggs continues
The moment a classical statistician or computer scientist creates an ad hoc model, probability is born.
Well, yes and no. We need simplifying models, but I can just observe frequencies as well to talk about probability.
Continuing
This is why frequentists, and even Bayesians and machine learners, speak of "learning" parameters (which must also exist and have "true" values), and of knowledge of "the true distribution"
What the krakenstician is not telling you, is that assumptions are done so any work can be done and are always open to error and revision in science. Assumptions should also be checked when possible. Assuming a parameter or model, including ones based on past evidence, is not done in any absolute Truth with a capital T religious sense. Rejection of a model is also open to error, and there are Type I, Type II, and other errors.
Those that practice krakenstats like to tilt at the 'parameters don't exist in reality' windmill, to try and knock down standard statistics, but this too is simple to grasp. For example, consider a parameter as a value from a population (a population we seek to learn about by taking a random sample). Say our population is all people in the United States, and we are looking at salaries, weights, or population count. Well, because salaries, weights, and people exist in reality, then sum(salaries), sum(weights), and sum(people count) exist. In fact, at a given time t, these are all constants.
Why Briggs would conclude that in this case a statistician would conclude the model is good for predictions only from a small p-value here is beyond me. The paper explicitly mentions that in the expansion of α, after Xtrain is exhausted, then the remaining digits are random. Briggs conveniently leaves out that the practice of statistics is using more than p-values (or any other single statistic). There is always guidance to look at a variety of things. A quick check of the statistics, assumptions, and graphs would show that overfitting was done. As usual, showing an abuse of a method does not show the method itself is wrong. Using hypothesis testing correctly here would actually help detect overfitting and help to create a better model.
Briggs wonders
Who needs to fit a model anyway, unless you want to make predictions with it?
Prediction for many time periods out is one use of a model, sure, but one could fit a model to describe/explain, as well as to get estimates of population parameters, be used to interpolate data, or just predict for one time period out. A model can also just be used to protect respondent data, in the case of sample surveys.
I can't help but observe over the years that folks like the krakenstician tend to dislike any results from science and statistics that have evidence against their religious and other beliefs (COVID-19 and vaccination data, election 2020 fraud conspiracy theory, global warming denial). For example, if a method uses hypothesis testing, parameters, experts, modelling, peer review, etc., then they will stretch to any length to try and cast hypothesis testing, parameters, experts, modelling, peer review, etc., as wrong...except when conclusions from using hypothesis testing, parameters, experts, modelling, peer review, etc., aligns with their beliefs.
Thanks for reading.
Please anonymously VOTE on the content you have just read:
Like:Dislike: